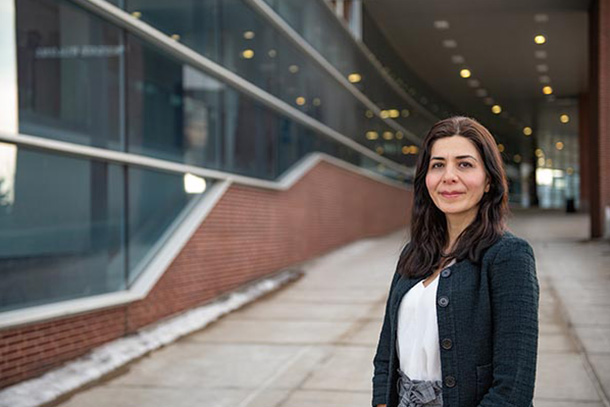
Parisa Shokouhi, Penn State professor of engineering science and mechanics, will build a physics-informed analysis and modeling framework that addresses the shortcomings in acoustic sensing data. Credit: Kelby Hochreither/Penn State
Parisa Shokouhi awarded NSF mid-career advancement grant
August 24, 2022
By Mariah Chuprinski
UNIVERSITY PARK, Pa. — Parisa Shokouhi, Penn State professor of engineering science and mechanics, was recently awarded a $360,000, three-year mid-career advancement grant from the National Science Foundation to develop a physics-informed machine learning framework that uses ultrasonic and acoustic data to characterize materials or predict failures.
Shokouhi’s research involves using acoustics in industrial and geophysical applications, such as using ultrasonic waves to conduct nondestructive evaluations of materials or predict failures in rock through ultrasonic and acoustic monitoring. She will use the new funding to develop algorithms to analyze ultrasonic and acoustic data to better characterize materials and predict failures. She also will create a graduate-level course on the subject.
“One of the objectives of the mid-career grant is to provide protected time for the principal investigator to explore a new direction, something beyond their main research focus,” Shokouhi said. “I am using the resources to dive deeper into data analytics and to expand my knowledge of the subject so I can train future computer scientists and engineers alike.”
To build and test the physics-informed machine learning framework, Shokouhi will use sample laboratory data from ultrasound transducers and arrays used for materials evaluation and monitoring. Her team will infuse physics into the algorithms so that the assessment or prediction does not deviate from the fundamental physical rules that make up the problem, a shortcoming of models that utilize data alone, according to Shokouhi.
“We plan to make the algorithm generalizable enough that the models can be applied in other circumstances,” Shokouhi said. “Since physics is common among different datasets, adding physics to machine learning makes the models more adaptable. Without adaptability, a model created for one dataset will perform poorly on another dataset even if the datasets are only slightly different.”
According to Shokouhi, the approach can provide just as accurate predictions with smaller datasets, which offers a practical advantage because large datasets are not always available nor accessible.
“This can significantly expand the application of machine learning-based modeling approaches to applications that use acoustic sensory data, such as industrial ultrasonic evaluation and monitoring, medical ultrasonic screening, building health diagnostics, and earthquake, mine and geothermal energy reservoir monitoring,” Shokouhi said.
Daniel Kifer, professor of computer science and engineering at Penn State, has an advisory role in this project.