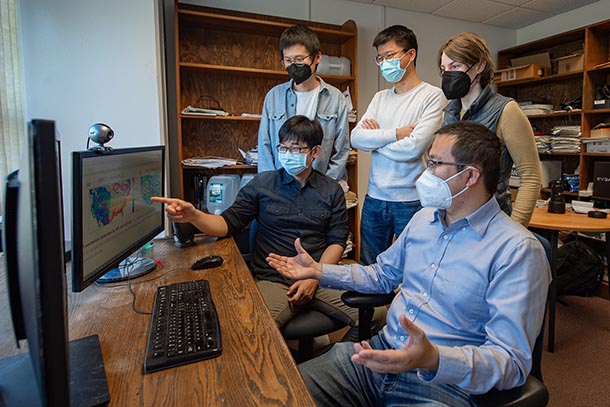
Chaopeng Shen, associate professor of civil and environmental engineering at Penn State (seated, right), and other researchers in his laboratory study a map that displays information that was derived from their new geoscientific modeling tool. Credit: Kelby Hochreither/Penn State.
New geoscientific modeling tool gives more holistic results in predictions
3/3/2022
By Sarah Small
UNIVERSITY PARK, Pa. — Geoscientific models allow researchers to test potential scenarios with numerical representations of the Earth and relevant systems, from predicting large-scale climate change effects to helping inform land management practices. Estimating parameters for traditional models, however, is computationally costly and calculates results for specific locations and scenarios that are difficult to extrapolate out to other scenarios well, according to Chaopeng Shen, associate professor of civil and environmental engineering at Penn State.
To address these issues, Shen and other researchers have developed a new model known as differentiable parameter learning that combines elements of both the traditional process-based models and machine learning for a method that can be applied broadly and lead to more aggregated solutions. Their model, published in Nature Communications, is publicly available for researchers to use.
“A problem that traditional process-based models face has been that they all need some kind of parameters — the variables in the equation that describe certain attributes of the geophysical system, such as such as conductivity of an aquifer or rainwater runoff — that they don’t have direct observations for,” Shen said. “Normally, you’d have to go through this process called parameter inversion or parameter estimation where you have some observations of the variables that the models are going to predict and then you go back and ask, ‘What should be my parameter?’”
A common process-based model is an evolutionary algorithm, which evolves across many iterations of operating so that it can better tune the parameters. These algorithms, however, are not able to handle large scales or be generalized to other contexts.
“It’s like I’m trying to fix my house, and my neighbor has a similar problem and is trying to fix his house, and there’s no communication between us,” Shen said. “Everyone is trying to do their own thing. Likewise, when you apply evolutionary algorithms to an area — let’s say to the United States — you will solve a separate problem for every little piece of land, and there’s no communication between them, so there is a lot of effort wasted. Further, everyone can solve their problem in their own inconsistent ways, and that introduces lots of physical unrealism.”
To solve issues for wider regions, Shen’s model takes in the data from all locations to get one solution. Instead of inputting location A data and getting location A solution, then inputting location B data for location B’s solution, Shen inputs locations A and B data for one solution that is more comprehensive.
“Our algorithm is much more holistic, because we use a global loss function,” he said. “This means that during the parameter estimation process, every location’s loss function — the discrepancy between the output of your model and the observations — is aggregated together. The problems are solved together at the same time. I’m looking for one solution to the entire continent. And when you bring more data points into this workflow, everyone is getting better results. While there were also some other methods that used a global loss function, humans were deriving the formula, so the results were not optimal. ”
Shen also noted that his method is much more computationally cost-effective than the traditional methods. What would normally take a super cluster of 100 processors two to three days now requires only one graphical processing unit one hour.
“The cost per grid cell dropped enormously,” he said. “It’s like economies of scale. If you have one factory that builds one car, but now you have the same one factory build 10,000 cars, your cost per unit declines dramatically. And that same thing happens as you bring more points into this workflow. At the same time, every location is now getting better service as a result of other locations’ participation.”
Pure machine learning methods can make good predictions for extensively observed variables, but they can produce results that are difficult to interpret because they do not include causal relationship assessment.
“A deep learning model might make a good prediction, but we don’t know how it did it,” Shen said, explaining that while a model may do a good job making predictions, researchers can misinterpret the apparent causal relationship. “With our approach, we are able to organically link process-based models and machine learning at a fundamental level to leverage all the benefits of machine learning and also the insights that come from the physical side.”
Other authors of the paper are: graduate students Dapeng Feng and Jiangtao Liu, postdoctoral scholar Wen-Ping Tsai and research associate Kathryn Lawson, all of the Penn State Department of Civil and Environmental Engineering; Ming Pan of the Scripps Institution of Oceanography at the University of California San Diego; Hylke Beck of GloH20, Almere, the Netherlands; and Yuan Yang of Tsinghua University and China Three Gorges Corporation, both of Beijing, China.
The U.S. Department of Energy and the National Science Foundation funded the research.