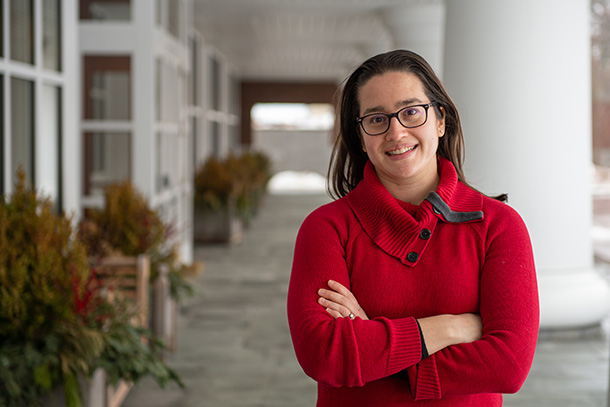
In two recent papers, Ilgin Guler, Penn State assistant professor of civil and environmental engineering, used statistical modeling and machine learning to monitor and forecast the conditions of 25,000 state-owned bridges in Pennsylvania. Many of them are structurally deficient. Credit: Kelby Hochreither/Penn State. All Rights Reserved.
Bridging the gap to better infrastructure
Study compares models for predicting bridge deterioration
June 29, 2022
By Mariah Chuprinski
UNIVERSITY PARK, Pa. — Bridge failures are a reality for states with aging infrastructure, including Pennsylvania. In February, the Forbes Avenue Bridge in Pittsburgh collapsed due to structural failure; unfortunately, this kind of event isn’t unique. According to the American Road and Transportation Builders Association, about 14% of Pennsylvania’s bridges are structurally deficient, meaning at least one of their key structural elements is in poor condition.
In two recent papers, Ilgin Guler, Penn State assistant professor of civil and environmental engineering, compared statistical modeling and machine learning to monitor and forecast the conditions of bridge decks, the topmost surfaces of a bridge. Her results are published in the Journal of Bridge Engineering and the Transportation Research Record.
“Both methods have their advantages,” Guler said. “The statistical method provides insights on the factors that contribute more or less to a bridge’s deterioration, while the machine learning method offers a more accurate prediction.”
For their dataset, researchers used a list of 25,000 state-owned bridges in Pennsylvania.
In the Journal of Bridge Engineering, researchers used an estimation method called the Markov chain Monte Carlo to write code in Python to represent the bridge data in statistical modeling. Then, they identified the coefficients or parameters, which told them everything they needed to know about the data, according to researchers.
“In the statistical distribution method, we were able to analyze explanatory variables, or the future impacts of different characteristics of the bridge, such as the types of materials, beams and rebars used to build the bridge,” said Muyang Lu, a Penn State doctoral student in civil and environmental engineering and first author on both papers. “The data address how likely it is that a factor may contribute to a bridge’s deterioration.”
In the Transportation Research Record paper, researchers used a machine learning method known as random survival forest, which creates data trees by splitting items into similar groups for analysis.
“Using machine learning, we created a prediction model using the 25,000-item dataset,” Guler said. “Over time, we trained the algorithm to ‘learn’ from the existing data to make assumptions about similar data.”
Machine learning methods have been used in the past to determine bridge deterioration for a given year, according to Guler, but they have not been used to predict the duration a bridge will stay in a certain condition or how long it will take to deteriorate.
“Both these methods can improve decision-making when stakeholders are designing bridges or deciding whether to make repairs and when,” Guler said. “With these data-driven models, we have a better estimate of what will deteriorate and when. This can drastically improve how bridge management is done.”
In addition to Guler and Lu, Aleksandra Radlińska, the Penn State Hartz Family Career Development Associate Professor of Civil and Environmental Engineering, and Jonathan Hydock, a Penn State undergraduate student in civil and environmental engineering, contributed to the Journal of Bridge Engineering paper.
The Center for Integrated Asset Management for Multimodal Transportation Infrastructure Systems: Region 3 University Transportation Center supported this work.