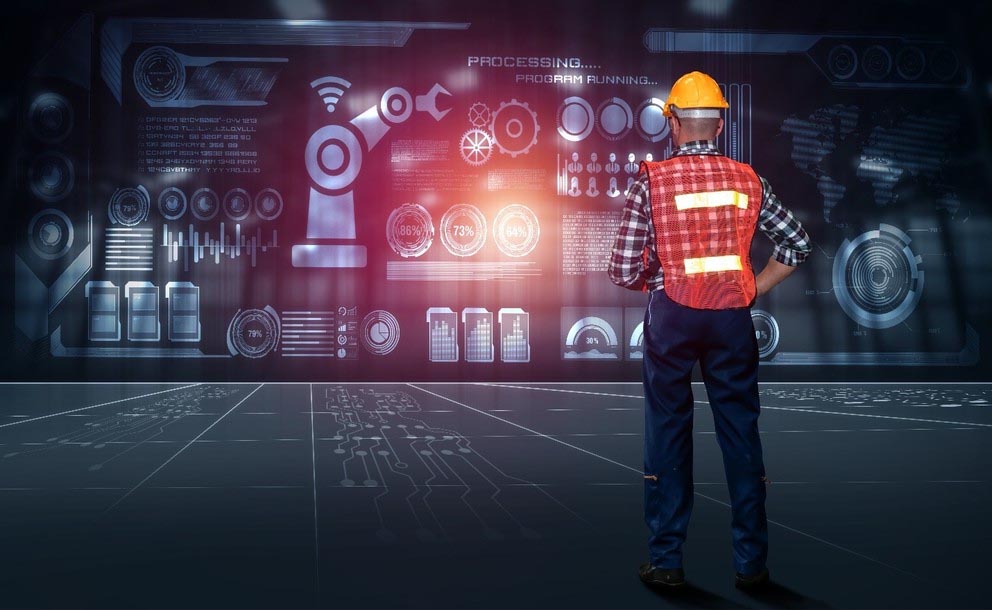
A construction worker in a worker-centered human robot environment. IMAGE: ADOBE STOCK, BLUE PLANET STUDIO
Worker-centered human robot partnerships -- a new chapter in construction
4/29/2021
By Pooja Nerkar
UNIVERSITY PARK, Pa. – In the future, humans may interact with artificially intelligent heavy machines, self-optimizing collaborative robots, unmanned terrestrial and aerial vehicles, and other autonomous systems, according to a team of Penn State engineers.
With the help of humans, these intelligent robots could perform strenuous and repetitive physical activities such as lifting heavy objects, delivering materials to the workers, monitoring the progress of construction projects, tying rebars, or laying bricks to build masonry walls.
However, this partnership can pose new safety challenges to workers, especially in the unstructured and dynamic environments of construction sites.
To a robot, a human operator is an unfailing partner. To a human, the robot’s level of awareness, intelligence and motorized precision can substantially deviate from reality, leading to unbalanced trust.
This creates a need for a change in designing collaborative construction robots toward ones that can monitor workers’ mental and physical stress and subsequently adjust their performance, according to Houtan Jebelli, assistant professor of architectural engineering.
Robots on construction sites are different from other industrial robots because they need to operate in highly fragmented and rugged workspaces with different layouts and equipment. In these environments, safe and successful delivery of work is not possible without human intervention, according to Jebelli.
This research on human-robot collaboration makes possible interaction between human and construction robots using brainwaves as indicators of workers’ mental activity. It is the first of its kind to integrate this technology with human-robot adaptation. The perceptual cues obtained from the brainwaves can also be used to develop a brain-computer interface approach (BCI) to create “hands-free” communication between construction robots and humans, mitigating the limitations of traditional robot control systems in other industries, said Jebelli.
“One novel aspect of the project to me is that it is one of the very first studies that try to measure and quantify workers’ cognitive load continuously, in near-real-time based on their physiological responses," said Jebelli. “Simultaneously, these decoded signals will be transferred into the robot’s motion planner for change of action.
“Once we capture workers’ cognitive load, we try to transfer this information into the robot so that the collaborative robot can monitor workers’ cognitive load,” Jebelli added.
Whenever the cognitive load is recognized to be higher than a specific threshold, the robot will reduce its pace to provide a safer environment for the workers, said Jebelli. This response could help design a collaborative robotic system that understands the human partner’s mental state and hopefully improve workers’ safety and productivity in the long term. The team published their results in two papers in Automation in Construction.
They also proposed a BCI-based system to operate a robot remotely.
“The ability to control a robot by merely imagining the commands can open new avenues to designing hands-free robotic systems in hazardous environments where humans require their hands to retain their balance and perform an action,” said Mahmoud Habibnezhad, a postdoctoral fellow conducting research with Jebelli.
The researchers capture workers’ brainwave signals with a wearable electroencephalogram (EEG) device and convert these signals into robotic commands.
“In our research, first we trained the subjects with a multiple imagery experiment,” said Yizhi Liu, doctoral student of architectural engineering. “The signal is then collected through EEG sensors and a spatial feature extraction technique called a common spatial pattern,” said Liu.
He explained that participants view images of specific actions, such as workers grabbing bricks with their right hands, and then imagine these actions. For example, when a subject imagines their right hand grabbing something, the right cortex of their brain generates a higher EEG signal than their left-brain area. The researchers employed machine learning to train the robots, using participants’ thoughts when imagining the actions. Subsequently, these translated signals will be transferred as digital commands to the robots through ROS, or robotic operating system, Liu added.
For the BCI system to continuously interpret brainwave signals from workers in near real-time, the researchers used three key elements — a wearable EEG device, a signal-interpretation application program interface (API), and a cloud server. The wearable EEG device captures the brainwave signals and sends them to the cloud server, and then the API begins generating commands.
The researchers created a network of channels between workers’ wearable biosensors and robots using ROS that acts as the middleware connecting different systems. Through these channels, commands such as the right-hand movement, left-hand movement and stop signal, can be easily sent to the robot. However, more nuanced commands require more data and improve the performance of the system and teleoperation of the robot, according to Jebelli.
“We developed a brain-computer interface system, which we can think of as a person trying to learn a new language that doesn’t know how to generate commands,” he said. “We try to connect different commands with some predefined patterns of their brainwaves.”
With more commands, the researchers can train and improve the performance of the system, according to Jebelli. These different commands include tasks such as controlling the robot, stopping the robot, or designing some predesigned work plan, such as delivering material from point A to point B by thinking about some specific tasks in the dictionary.
“This is a framework that we tested out for one robot, that is a proof-of-concept that the framework is working,” said Habibnezhad. “We can improve the framework by using different robots or drones or different systems. We can improve the accuracy of the control by using more commands and trying to extract more patterns and defining different controls.”