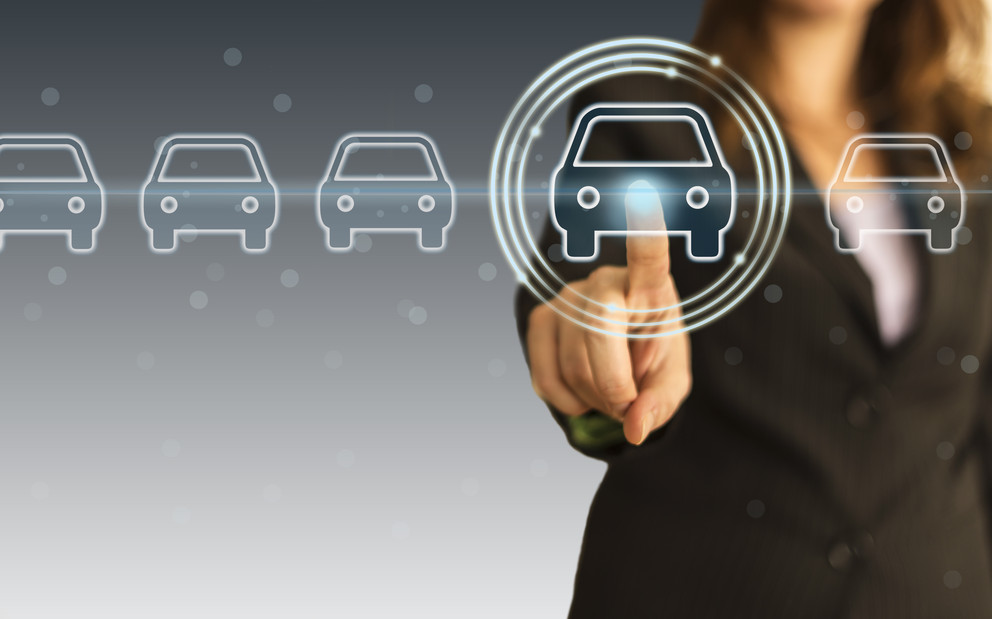
Behind the scenes, algorithms help decide what kinds of vehicle options come to market based upon consumer opinions. IMAGE: iStock/@fatido
Driving robust decision making: General Motors seeks academic expertise
9/14/2020
By Miranda Buckheit
UNIVERSITY PARK, Pa. — In the automotive industry, content decisions ranging from engine selection to the style of seats can affect a vehicle’s market sales. Automakers need to anticipate vehicle trims and options that customers will want to purchase from dealer lots. Failing to deliver the preferred combinations of content features may cause General Motors (GM) to lose customers to competitors or experience delayed purchases.
To help avoid such issues, GM has developed a vehicle content optimization (VCO) capability to help provide the right content at the right price to the market.
However, something was missing for the VCO — the market simulator in which it was applied had too much uncertainty.
To measure and reduce uncertainty in GM’s vehicle market simulator to improve GM’s VCO, GM project manager and technical fellow Peiling Wu-Smith partnered with Eunhye Song, Harold and Inge Marcus Early Career Assistant Professor of Industrial and Manufacturing Engineering at Penn State, and Barry L. Nelson, Walter P. Murphy Professor of Industrial Engineering and Management Sciences at Northwestern University.
“VCO is an important part of the GM vehicle development process to help GM improve its market share and profitability,” Wu-Smith said. “We were initially using point estimates in the VCO as vehicle program teams prefer that ways. However, we recognized the potential risk of not modeling uncertainty and sought collaboration with Professors Nelson and Song to explore that.”
Dealers stock their lots by ordering vehicles with different content available in GM’s content portfolio, which is the complete set of combinations offered for a particular vehicle model. This makes it imperative to reflect customers’ preferences within the portfolio, according to the company.
Conversely, when collecting information about consumer preferences, companies may not always have the exact answers. GM performs market research surveys for each vehicle model to learn customers’ preferences, but the sample size does not always accurately reflect the preferences of the larger market. This creates a discrepancy between the model constructed from the survey data and market preferences.
“Content optimization is the process of deciding what features to include in a vehicle — do it well and you sell more but do it badly and the vehicle can be a flop,” Nelson said. “Billions of dollars are at stake, but recommendations based on a mathematical-computer model are always subject to risk because models are not perfect.”
Uncertainty quantification, which measures how variable the model outcomes are considering uncertainties about the model inputs, plays a key role in reaching a robust business decision via computational simulation, said Song.
“The VCO can predict what sales would be for their vehicle models three to five years in the future, which is a high-stakes situation that has various usage for GM internally, so it’s important to provide prediction error reflecting uncertainty in the model,” Song said. “Uncertainty quantification needs orders-of-magnitude higher computation power, and they didn’t know where to start, so they wanted to come up with a feasible plan to measure uncertainties for their model.”
To do so, Song and the team aimed to establish an uncertainty quantification procedure for the VCO that works on any GM vehicle model. They report their results in the Journal of Applied Analytics.
Song and the team began with identifying the key inputs to GM’s market simulator, which induce uncertainty in the simulation outcomes. Customer preference reflecting market research survey results is one of many key inputs. From this information, the model uses virtual customers to predict market outcomes: sales volume, revenue and market share.
“Before you run the simulator, you need to calibrate the simulator with parameters that reflect the market and sales data,” Song said. “This is the most computationally demanding part, as it is a stochastic optimization problem without a known mathematical structure. Before we joined the project, GM ran a new calibration every time a new simulation run was made, which made it computationally expensive to run multiple runs of simulation for uncertainty quantification. As a result of uncertainty quantification, we discovered that calibration needs to be done only once for each vehicle model.”
According to Song, consumer utility is a major contributor to uncertainty in the simulation outcomes; however, with uncertainty quantification, GM can uncover crucial insights into how confident GM can be in setting direction for the years to come.
“Uncertainty quantification helps them make big decisions because when they compare many candidate portfolios to choose one, they can put confidence bands around predicted sales volumes instead of just comparing the point estimates,” Song said.
Additionally, they worked to provide actionable guidance on how to efficiently reduce uncertainty in their estimates, which would allow GM to decrease estimation error to make more robust business decisions.
“Some risks can be reduced, but others are inherent,” Nelson said. “Our work helps GM reduce risks where they can, and hedge against risks that they can’t reduce.”
This work was funded by GM during Song’s doctoral studies at Northwestern University. Song continued the project as a faculty member of the Penn State Harold and Inge Marcus Department of Industrial and Manufacturing Engineering.